Unleashing the power of data observability: from ingestion to destination
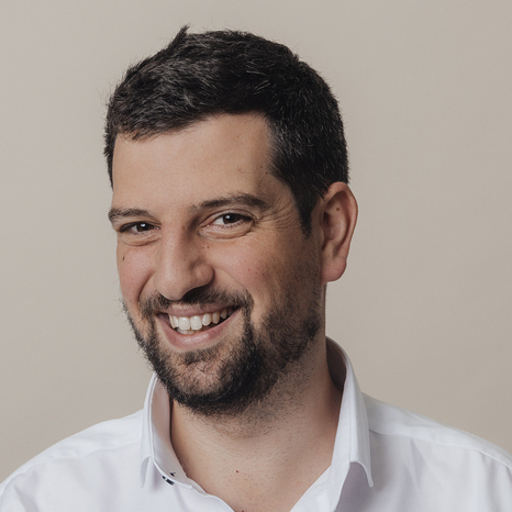

Trust is the cornerstone of effective data operations. But in order to trust data, people need confidence that the data is correct.
As data pipelines grow in complexity, maintaining this trust becomes challenging. That’s where data observability comes in.
Data observability is more than just a buzzword; it's a powerful capability that enables teams to see, understand and trust their data at every stage of its journey.
This post explores the nuances of data observability, why it’s vital in modern data operations and how shifting to a platform approach can reinforce trust within organizations.
What is data observability?
Data observability is the ability to understand the health and reliability of data systems, processes, and pipelines. It offers insights into data quality, integrity, and lineage, making it easier to detect, diagnose, and resolve issues that could impact downstream analytics and decision-making.
Unlike traditional data monitoring, which focuses on system metrics like CPU usage, data observability dives deep into the data itself. It identifies anomalies, detects schema changes, and tracks lineage, giving data teams the insights they need to address issues early.
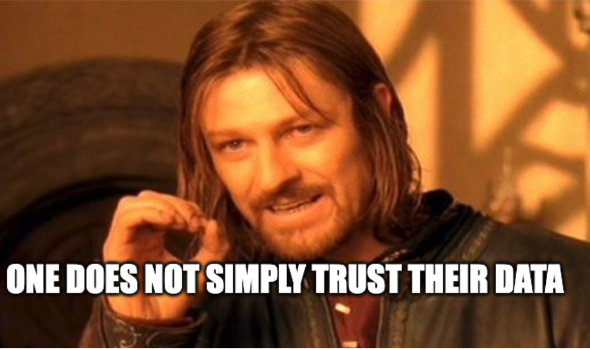
Why data observability matters for today’s data ecosystem
Modern data ecosystems are complex, involving multiple data sources, transformations, warehouses, and destinations. Data observability plays a critical role in ensuring that every component in this ecosystem functions as expected so everyone can trust the data.
- Proactive Issue Detection: Observability provides visibility into the data flow, enabling data teams to identify and address issues before they cause major disruptions.
- Data Quality Assurance for AI and More: By continuously monitoring the data, observability ensures high data quality, which translates to better insights and decisions. This is essentially for companies leveraging their data to create ML models and for AI applications.
- Efficiency for Data Engineers: With observability embedded into each stage, engineers spend less time troubleshooting and more time driving strategic initiatives.
For organizations that depend on accurate data to fuel their business intelligence and AI, data observability has become indispensable. It builds a foundation of trust in data, which is critical for scaling analytics and insights.
Data observability in Matia: the platform approach
Matia’s data observability isn’t confined to just one segment of the data pipeline. It’s embedded at every stage, providing a seamless layer of transparency and control from the moment raw data is generated and until it reaches its final destination. With Matia, monitoring and observability can 'shift left,' allowing critical anomaly detection to happen earlier in data pipeline development. Here’s a breakdown of how Matia ensures data observability across data pipelines:
1. At the source: database observability
Matia maintains a vigilant watch over your transactional databases, analyzing data patterns and detecting anomalies in real-time. By establishing baseline metrics and leveraging statistical models, Matia can identify unexpected changes in data volume, unusual value distributions, or deviations from historical patterns within databases like Postgres and MySQL. This proactive monitoring helps teams catch data quality issues at their source, ensuring problems are detected before they can cascade through your data pipeline.
2. During ingestion: real-time monitoring
As data flows into Matia’s ingestion layer, it’s continuously monitored for consistency, format compliance, and other quality metrics. By embedding observability directly within the ingestion process, Matia provides an additional layer of assurance, helping teams to proactively address any ingestion issues before data reaches the transformation stage. Additionally, monitoring data quality during ingestion means that Matia is able to save on data warehouse queries downstream, reducing users’ infrastructure costs.
3. At the warehouse or data lake: Snowflake and Databricks observability
After ingestion, data lands in a warehouse or data lake where it often undergoes transformations. Matia’s observability tools provide visibility into transformation results, ensuring that each transformation meets expectations and maintains data integrity. By monitoring transformations, Matia empowers data engineers to validate complex ETL processes and mitigate potential data quality issues as they arise.
4. On transformation outputs: ensuring quality post-transformation
Transformations are where the magic of data processing often happens, but they can also introduce errors. With Matia’s observability, every transformation output is scrutinized, making sure that the data is not only transformed accurately but also maintains the necessary quality standards for downstream use.
Matia offers a deep integration with dbt, surfacing model run results and errors metadata, full lineage ERDs (those diagrams showing which of your tables are BFFs) and a comprehensive view of your dbt tests, along with historical view of past dbt test results.
5. The RETL model: observability in reverse ETL
Matia’s RETL (Reverse ETL) processes allow data teams to push insights back to operational tools and systems, like CRMs or marketing platforms. Observability ensures that the data pushed back is complete, timely, and error-free. This capability is essential for data-driven organizations that rely on real-time data to drive customer interactions, personalize experiences, and automate decisions.
While Matia is not an orchestration platform, users can also sequence their RETL to sync after their dbt model runs.
The Matia advantage: full-pipeline observability
Matia’s unique approach to observability is a game-changer for data teams. Instead of patching issues after the fact, Matia provides continuous visibility, catching and correcting errors as they arise throughout the data pipeline and stopping faulty data from being pushed to downstream tools. Matia’s proactive “shift-left” approach catches problems before they impact end users, ensuring data remains reliable, trustworthy, and actionable throughout the entire pipeline. By embedding observability across each stage, Matia empowers organizations and supercharges data teams in numerous ways:
- Reduce Data Downtime: With fewer data issues, analytics and insights are available when they’re needed most
- Increase Confidence in Data: Observability fosters trust, ensuring everyone know they’re working with accurate, reliable data. It can even alert stakeholders when you know there’s going to be an anomaly before they look at their dashboard and complain
- Enhance Operational Efficiency: Less troubleshooting frees up data engineers to focus on high-impact work
Matia’s approach to data observability equips organizations with the visibility they need to trust and act on their data. Additionally, customers can receive multi channel alerts when something doesn’t go as expected.
Why choose Matia for your data observability needs?
Data observability isn’t just about monitoring: it’s about creating trust in your data and empowering data teams to stay ahead of issues, maintain data quality, build reliable AI models and drive smarter decisions. Matia’s platform offers a comprehensive, end-to-end observability solution that provides data teams with peace of mind, from the moment data enters the pipeline to its final destination, without the headache of having to choose other tools
In a world where data reliability and accessibility are paramount, Matia delivers the tools and insights needed to transform observability from a reactive process to a proactive advantage.